Revisiting Consistency Regularization for Semi-Supervised Learning
Article Ecrit par: Kukleva, Anna ; Dai, Dengxin ; Schiele, Bernt ; Fan, Yue ;
Résumé: Consistency regularization is one of the most widely-used techniques for semi-supervised learning (SSL). Generally, the aim is to train a model that is invariant to various data augmentations. In this paper, we revisit this idea and find that enforcing invariance by decreasing distances between features from differently augmented images leads to improved performance. However, encouraging equivariance instead, by increasing the feature distance, further improves performance. To this end, we propose an improved consistency regularization framework by a simple yet effective technique, FeatDistLoss, that imposes consistency and equivariance on the classifier and the feature level, respectively. Experimental results show that our model defines a new state of the art across a variety of standard semi-supervised learning benchmarks as well as imbalanced semi-supervised learning benchmarks. Particularly, we outperform previous work by a significant margin in low data regimes and at large imbalance ratios. Extensive experiments are conducted to analyze the method, and the code will be published.
Langue:
Anglais
Thème
Informatique
Mots clés:
Classification
Semi-supervised learning
Representation learning
Consistency regularization
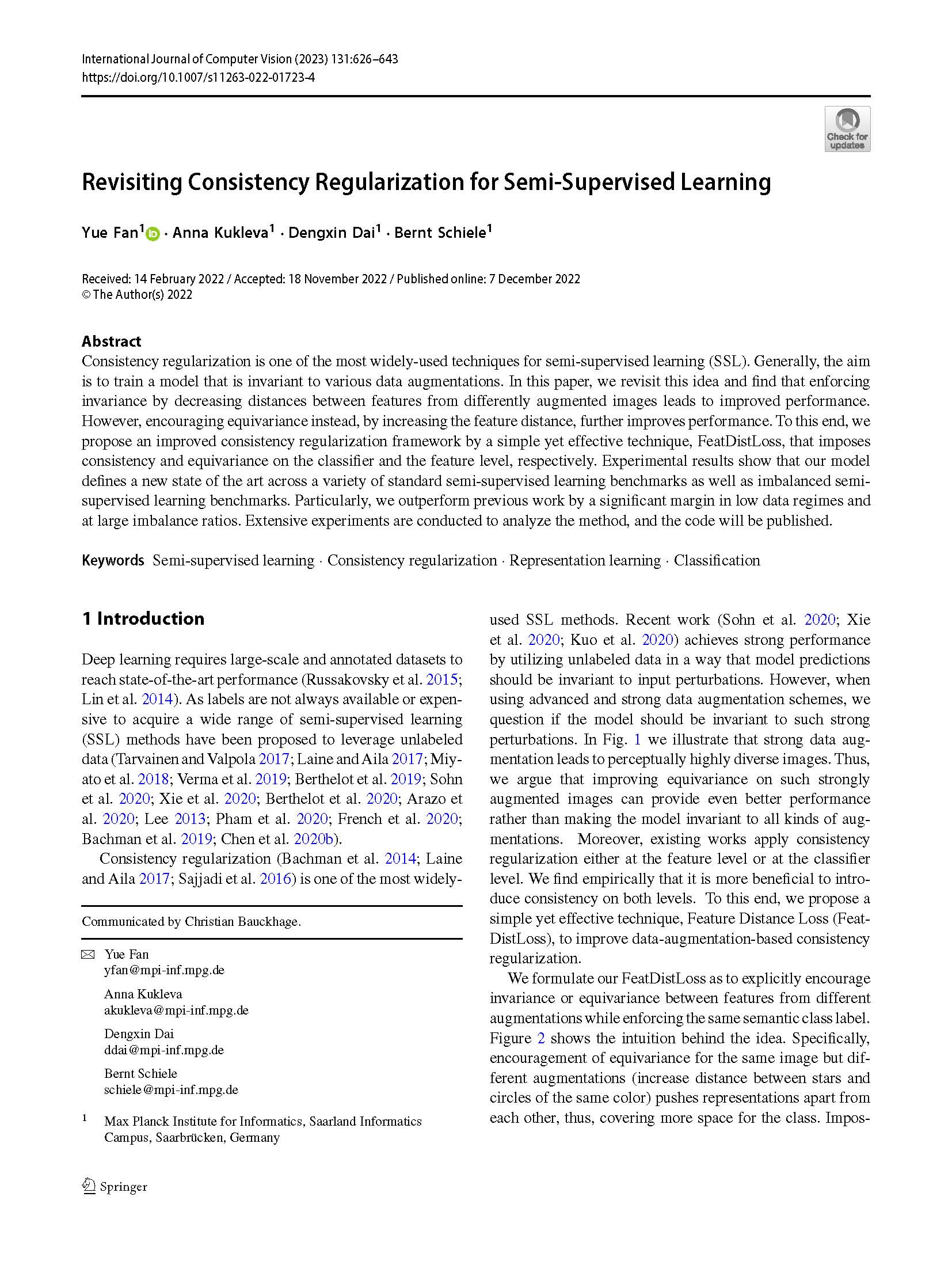